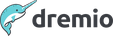
Dremio
Founded Year
2015Stage
Series E | AliveTotal Raised
$405MValuation
$0000Last Raised
$160M | 3 yrs agoMosaic Score The Mosaic Score is an algorithm that measures the overall financial health and market potential of private companies.
-24 points in the past 30 days
About Dremio
Dremio operates in the data management industry, providing a platform for self-service analytics and AI. The company offers tools including a SQL query engine and lakehouse management, along with various connectors and integrations for data analysis. Dremio serves sectors that require data analytics and management solutions, such as technology and finance. It was founded in 2015 and is based in Santa Clara, California.
Loading...
ESPs containing Dremio
The ESP matrix leverages data and analyst insight to identify and rank leading companies in a given technology landscape.
The generative AI — text-to-code & data querying market offers solutions that can automatically generate code from natural language descriptions. This technology can save time and increase efficiency for developers, as well as make coding more accessible to those without extensive programming knowledge. The market offers solutions that can transform the way we approach coding and software developm…
Dremio named as Highflier among 4 other companies, including Hugging Face, Seek AI, and Veezoo.
Loading...
Research containing Dremio
Get data-driven expert analysis from the CB Insights Intelligence Unit.
CB Insights Intelligence Analysts have mentioned Dremio in 5 CB Insights research briefs, most recently on Aug 4, 2023.
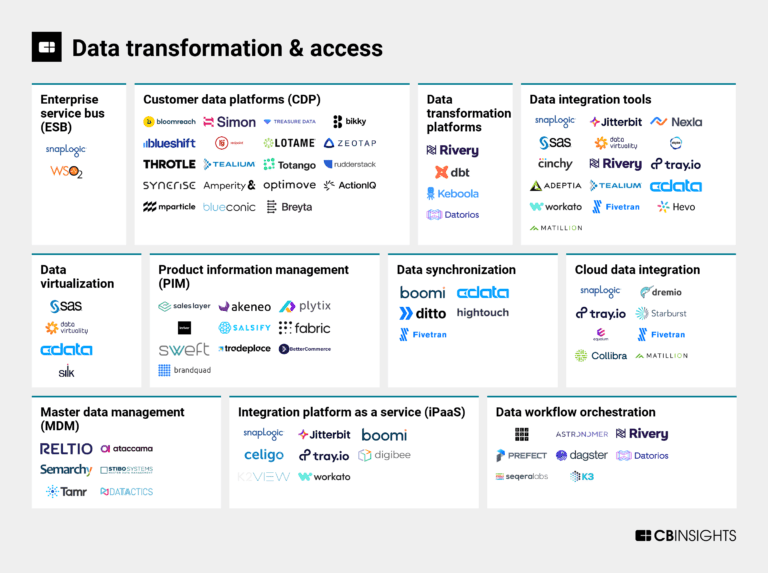
Aug 4, 2023
The data transformation & access market map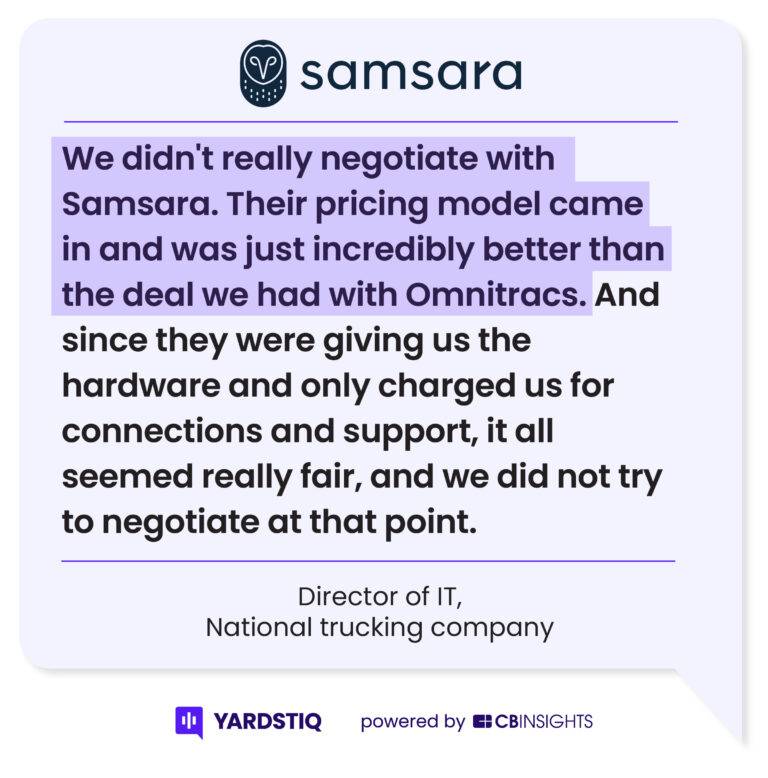
Oct 25, 2022
The Transcript from Yardstiq: Toppling SalesforceExpert Collections containing Dremio
Expert Collections are analyst-curated lists that highlight the companies you need to know in the most important technology spaces.
Dremio is included in 3 Expert Collections, including Unicorns- Billion Dollar Startups.
Unicorns- Billion Dollar Startups
1,257 items
AI 100
100 items
Artificial Intelligence
7,146 items
Dremio Patents
Dremio has filed 8 patents.
The 3 most popular patent topics include:
- data management
- database management systems
- planetary systems
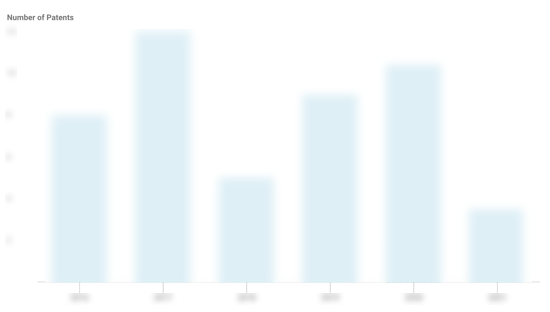
Application Date | Grant Date | Title | Related Topics | Status |
---|---|---|---|---|
10/8/2021 | 8/15/2023 | Database management systems, Data management, SQL, Relational database management systems, Planetary systems | Grant |
Application Date | 10/8/2021 |
---|---|
Grant Date | 8/15/2023 |
Title | |
Related Topics | Database management systems, Data management, SQL, Relational database management systems, Planetary systems |
Status | Grant |
Latest Dremio News
Jan 13, 2025
(bsd-studio/Shutterstock) The humble data lakehouse emerged about eight years ago as organizations sought a middle ground between the anything-goes messiness of data lakes and the locked-down fussiness of data warehouses. The architectural pattern attracted some followers, but the growth wasn’t spectacular. However, as we kick off 2025, the data lakehouse is poised to grow quite robustly, thanks to a confluence of factors. As the big data era dawned back in 2010, Hadoop was the hottest technology around, as it provided a way to build large clusters of inexpensive industry-standard X86 servers to store and process petabytes of data much more cheaply than the pricey data warehouses and appliances built on specialized hardware that came before them. By allowing customers to dump large amounts of semi-structured and unstructured data into a distributed file system, Hadoop clusters garnered them the nickname “data lakes.” Customers could process and transform the data for their particular analytical needs on-demand, or what’s called a “structure on read” approach. This was quite different than the “structure on write” approach used with the typical data warehouse of the day. Before Hadoop, customers would take the time to transform and clean their transactional data before loading it into the data warehouse. This was obviously more time-consuming and more expensive, but it was necessary to maximize the use of pricey storage and compute resources. As the Hadoop experiment progressed, many customers discovered that their data lakes had turned into data swamps. While dumping raw data into HDFS or S3 radically increased the amount of data they could retain, it came at the cost of lower quality data. Specifically, Hadoop lacked the controls that allowed customers to effectively manage their data, which led to lower trust in Hadoop analytics. By the mid-2010s, several independent teams were working on a solution. The first team was led by Vinoth Chandar, an engineer at Uber, who needed to solve the fast-moving file problem for the ride-sharing app. Chandar led the development of a table format that would allow Hadoop to process data more like a traditional database. He called it Hudi, which stood for Hadoop upserts, deletes, and incrementals. Uber deployed Hudi in 2016. A year later, two other teams launched similar solutions for HDFS and S3 data lakes. Netflix engineer Ryan Blue and Apple engineer Daniel Weeks worked together to create a table format called Iceberg that sought to bring ACID-like transaction capabilities and rollbacks to Apache Hive tables. The same year, Databricks launched Delta Lake, which melded the data structure capabilities of data warehouses with its cloud data lake to bring a “good, better, best” to data management and data quality. These three table formats largely drove the growth of data lakehouses, as they allowed traditional database data management techniques to be applied as a layer on top of Hadoop and S3-style data lakes. This gave customers the best of both worlds: The scalability and affordability of data lakes and the data quality and reliability of data warehouses. Other data platforms began adopting one of the table formats, including AWS , Google Cloud , and Snowflake . Iceberg, which became a top-level Apache project in 2020, garnered much of its traction from the open source Hadoop ecosystem. Databricks, which initially kept close tabs on Delta Lake and its underlying table format before gradually opening up, also became popular as the San Francisco-based company rapidly added customers. Hudi, which became a top-level Apache project in 2019, was the third most-popular format. The battle between Apache Iceberg and Delta Lake for table format dominance was at a stalemate. Then in June of 2024, Snowflake bolstered its support for Iceberg by launching a metadata catalog for Iceberg called Polaris (now Apache Polaris). A day later, Databricks responded by announcing the acquisition of Tabular , the Iceberg company founded by Blue, Weeks, and former Netflix engineer Jason Reid, for between $1 billion and $2 billion. Databricks executives announced that Iceberg and Delta Lake formats would be brought together over time. “We are going to lead the way with data compatibility so that you are no longer limited by which lakehouse format your data is in,” the executives, led by CEO Ali Ghodsi, said. Tabular CEO Ryan Blue (right) and Databricks CEO Ali Ghodsi on the stage at Data + AI Summit in June, 2024 The impact of the Polaris launch and Tabular acquisitions were huge, particularly for the community of vendors developing independent query engines, and it immediately drove an uptick in momentum behind Apache Iceberg. “If you’re in the Iceberg community, this is go time in terms of entering the next era,” Read Maloney, Dremio ’s chief marketing officer, told this publication last June. Seven months later, that momentum is still going strong. Last week, Dremio published a new report, titled “State of the Data Lakehouse in the AI Era,” which found growing support for data lakehouses (which are now considered to be Iceberg based, by default). “Our analysis reveals that data lakehouses have reached a critical adoption threshold, with 55% of organizations running the majority of their analytics on these platforms,” Dremio said in its report, which is based on a fourth-quarter survey of 563 data decision-makers by McKnight Consulting Group. “This figure is projected to reach 67% within the next three years according to respondents, indicating a clear shift in enterprise data strategy.” Dremio says that cost efficiency remains the primary driver behind the growth in data lakehouse, cited by 19% of respondents, followed by unified data access and enhanced ease of use (17% respectively) and self service analytics (13%). Dremio found that 41% of lakehouse users have migrated from cloud data warehouses and 23% have transitioned from standard data lakes. Better, more open data analytics is high on the list of reasons to move to a data lakehouse, but Dremio found a surprising number of customers using their data lakehouse to back another use case: AI development. The company found an astounding 85% of lakehouse users are currently using their warehouse to develop AI models, with another 11% stating in the survey that they planned to. That leaves a stunning 4% of lakehouse customers saying they have no plans to support AI development; it’s basically everyone. While AI aspirations are universal at this point, there are still big hurdles to overcome before organizations can actually achieve the AI dream. In its survey, Dremio found organizations reported serious challenges to achieving success with AI data prep. Specifically, 36% of respondents say governance and security for AI use cases is the top challenge, followed by high cost and complexity (cited by 33%) and a lack of a unified AI-ready infrastructure (20%). The lakehouse architecture is a key ingredient for creating data products that are well-governed and widely accessible, which are critical for enabling organizations to more easily develop AI apps, said James Rowland-Jones (JRJ), Dremio’s vice president of product management. “It’s how they share [the data] and what comes with it,” JRJ told BigDATAwire at the re:Invent conference last month. “How is that enriched. How do how do you understand it and reason over it as an end user? Do you get a statistical sample of the data? Can you get a feel for what that data is? Has it been documented? Is it governed? Is there a glossary? Is the glossary reusable across views so people aren’t duplicating all of that effort?” Dremio is perhaps best known for developing an open query engine, available under an Apache 2 license, that can run against a variety of different backends, including databases, HDFS, S3, and other file systems and object stores. But the company has been putting more effort lately into building a full lakehouse platform that can run anywhere, including on major clouds, on-prem, and in hybrid deployments. The company was an early backer of Iceberg with Project Nessie, its metadata catalog. In 2025, the company plans to put more focus on bolstering data governance, security, and building data products, company executives said at re:Invent. The biggest beneficiary of the rise of open, Iceberg-based lakehouse platforms are enterprises, who are no longer beholden to monolithic cloud platforms vendors that want to lock customers’ data in so they can extract more money from them. A side effect of the rise of lakehouses is that vendors like Dremio now have the ability to sell their wares to customers, who are free to pick and choose a query engine to meet their specific needs. “The data architecture landscape is at a pivotal point where the demands of AI and advanced analytics are transforming traditional approaches to data management,” Maloney said in a press release. “This report underscores how and why businesses are leveraging data lakehouses to drive innovation while addressing critical challenges like cost efficiency, governance, and AI readiness.” Related Items:
Dremio Frequently Asked Questions (FAQ)
When was Dremio founded?
Dremio was founded in 2015.
Where is Dremio's headquarters?
Dremio's headquarters is located at 3970 Freedom Circle, Santa Clara.
What is Dremio's latest funding round?
Dremio's latest funding round is Series E.
How much did Dremio raise?
Dremio raised a total of $405M.
Who are the investors of Dremio?
Investors of Dremio include Lightspeed Venture Partners, Norwest Venture Partners, Cisco Investments, Insight Partners, Sapphire Ventures and 7 more.
Who are Dremio's competitors?
Competitors of Dremio include CData, Onehouse, Keboola, Varada, Aiven and 7 more.
Loading...
Compare Dremio to Competitors
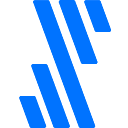
Fivetran specializes in automated data movement and focuses on data integration and ELT processes within the technology sector. The company offers a platform that extracts, loads, and transforms data from various sources into cloud data destinations, enabling efficient and reliable data centralization. Fivetran primarily serves sectors that require robust data analytics and operational efficiency, such as finance, marketing, sales, and support. It was founded in 2012 and is based in Oakland, California.

Adverity focuses on data management and operations. The company offers an integrated data platform that enables businesses to connect, manage, and use their data at scale, blending disparate datasets such as sales, marketing, and advertising. It primarily serves sectors such as marketing, engineering, and analytics. Adverity was founded in 2015 and is based in Vienna, Austria.
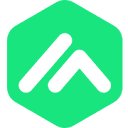
Matillion offers a productivity platform for data teams operating in the data management and cloud computing industries. The company offers services that enable both coders and non-coders to move, transform, and orchestrate data pipelines. Its primary customer segments include the financial services, healthcare, life sciences, retail, communications and media, and technology and software industries. It was founded in 2011 and is based in Manchester, United Kingdom.
Syncari is a company that provides data unification and automation, focusing on master data management (MDM) within the data management industry. The company offers a data management platform that allows for synchronization and unification of data across organizational systems. Syncari's solutions aim to assist in decision-making and data quality. It was founded in 2019 and is based in Newark, California.

Hightouch is a Composable Customer Data Platform (CDP) that specializes in data activation and reverse ETL services. The company offers a suite of products that enable businesses to sync data from their data warehouses to over 200 tools, build and manage customer profiles, and deliver personalized marketing campaigns without the need for coding. Hightouch primarily serves sectors such as retail and eCommerce, media and entertainment, financial services, healthcare, and B2B SaaS. It was founded in 2018 and is based in San Francisco, California.

Denodo specializes in data management, logical data management, and data virtualization. The company offers a platform that integrates, manages, and secures enterprise data from various sources, providing a unified access layer for analytical and operational use cases. Denodo's platform is designed to support AI initiatives, deliver real-time business intelligence, and enable self-service data democratization across multiple industries. It was founded in 1999 and is based in Palo Alto, California.
Loading...